The development of AI for real-time flood forecasting represents a transformative leap in disaster preparedness and response in Aotearoa New Zealand.
- Traditional physical flood models are computationally intensive and take up to 24 hours to run, limiting their utility in rapidly evolving flood scenarios.
- An AI model developed by NIWA runs in 1-2 minutes, providing near-instantaneous flood impact forecasts.
- Using the 2021 Buller River flood as a case study, NIWA’s AI model showed it could accurately predict flood depths and inundation areas with 95% accuracy.
When the Buller River broke its banks in July 2021, the flood flows were the highest recorded on any river, anywhere in the country since 1926. The inundation of Westport and surrounding areas resulted in 826 properties and 2,000 people being evacuated.
“Floods are New Zealand’s most frequent and most costly natural disaster. Fast and accurate flood impact forecasting is crucial for reducing the risk to life, property and infrastructure,” says Niwa Data Scientist Dr Deidre Cleland.
"Effective flood preparation and response requires detail beyond river flows. We need to forecast where it will flood, and when," she says.
While currently available physical prediction models that use weather, ocean and soil moisture data can help local authorities, residents, and farmers to prepare, these can take up to 24 hours to run, making them less useful in a rapidly unfolding flood event.
Using the Westport flood as a case study, Dr Deidre Cleland and her Niwa colleagues Dr Céline Cattoën-Gilbert, and Richard Measures used machine learning (ML) to create an AI flood prediction model that runs in 1-2 minutes – a fraction of the time.
The pre-trained model can forecast hourly flood inundation maps in real-time. The team trained the model using a library of Westport flood scenarios spanning the range of possible conditions.
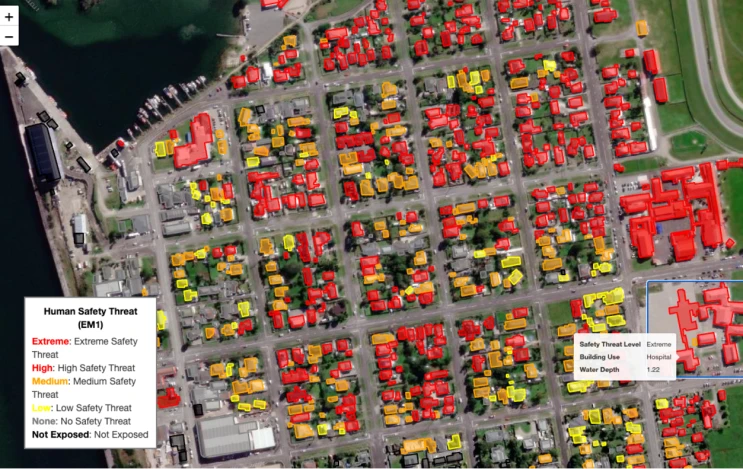
AI flood prediction visualisation - Human Safety Threat | Image credit: NIWA
“Each flood training simulation starts with a forecast for the sea level at the coast of Westport and the river flow down the Buller River,” says Dr Cleland.
A convolutional neural network, the model is trained to predict hourly flood maps from training simulations using only the sea level and river flow forecasts 8-12 hours prior.
Its accuracy was assessed by comparing it against simulations to flood maps forecast using a physical model. The ML model combines all the different layers of information it uses to make a prediction into a grid-like matrix of cells. When compared to the physical model, it’s average error in predicted flood depth was under 0.1 metres per cell, and the ML flood inundation area was forecast with an average accuracy of 95%.
“This process requires a lot of computing power. But by generating the library and training ahead of time, the ML model is ready to respond in real-time to an incoming weather event,” says Dr Cleland.
When NIWA makes a weather forecast it uses an ensemble of weather models to capture the inherent uncertainties in predicting wild weather likely to cause a flood.
Rather than relying on just one model to predict a flood, it uses a range of scenarios to consider small variations in rainfall, river levels and the amount of water in the soil.
The efficiency of the ML flood map model means that NIWA can now generate flood maps that predict the probability of flooding over time as well as the depth of the flood.
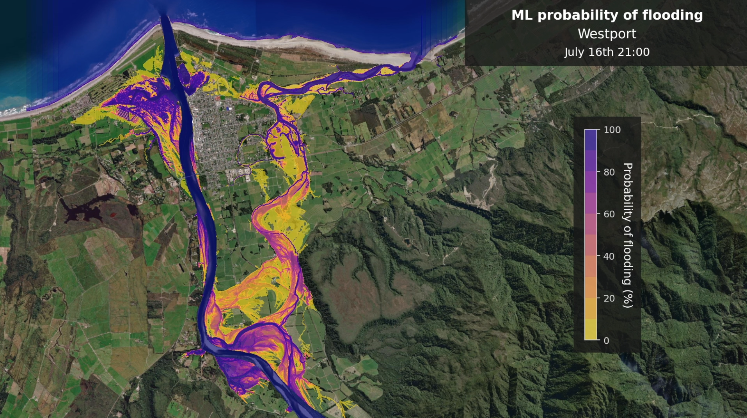
Flood risk visualisation - ML probability of flooding | Image credit: NIWA
“This means we can better understand the risk of flooding days ahead of an extreme weather event,” says Dr Cleland.
“Whether it’s cleaning out gutters, stocking up on sandbags, or planning for potential evacuations, the extra time gained by using real-time ML flood map forecasts will be crucial for flood preparedness.
“It will give emergency responders more time to make informed decisions and will help keep West Coast residents and their homes, property and businesses safer,” she says.
Looking ahead, Dr Cleland and team plan to operationalise the ML model so that rapid flood map forecasting is available for a real, incoming flood event in Westport.
“We are also working on extending the ML approach to other locations around New Zealand, starting with those at highest risk of flooding,” she says.